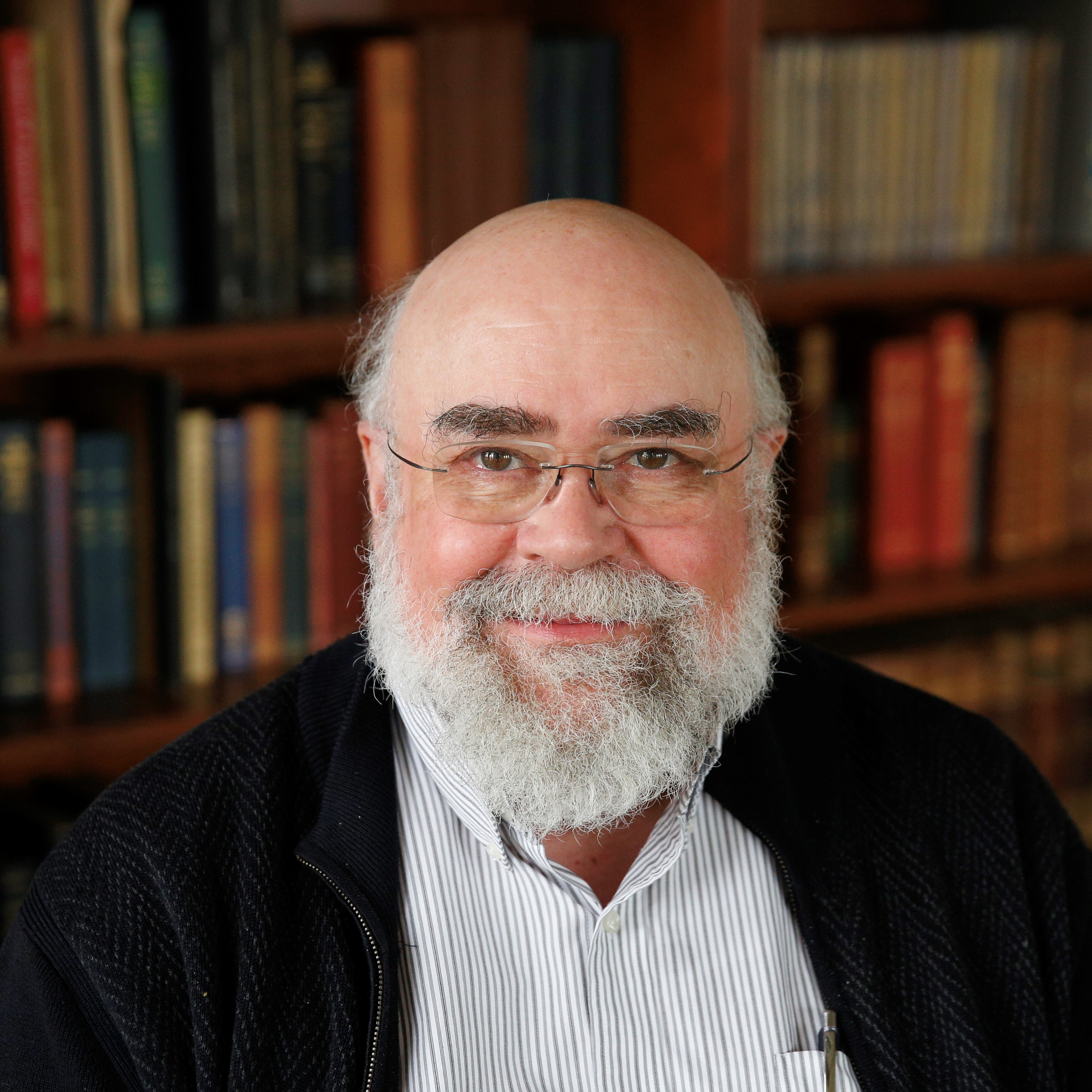
Regents Professor of History and Population Studies
Director, Institute for Social Research and Data Innovation
University of Miinnesota
ruggles@umn.edu
(612) 624-5818
Response to Hullman's Blog Post
This post responds to a blog post by Jessica Hullman discussing my recently-published research brief with David Van Riper.
Hullman has several complaints about our presentation. In particular, she says that our Monte-Carlo simulation was not well explained, and that she had to spend an inordinate amount of time figuring it out. I am sorry she had so much trouble. None of the seven external reviewers of the paper seem to have had any problem understanding our explanation of the model, and one of them replicated it in R. Perhaps this reflects differences in style and terminology between demography and computer science.
Hullman's main substantive point is that the database reconstruction performs better than chance when you focus on tiny blocks, those with fewer than 10 residents. We devote an entire section of our brief to this issue. As we point out, database reconstruction ought to work best with small blocks where the published tables directly reveal unique combinations of respondent characteristics. The key table powering the database reconstruction experiment--Summary File 1 P012A-I--provides information on age by sex by race by ethnicity. This table can easily be rearranged into individual-level format, providing the age, sex, and race/ethnicity of the population of each block with near-perfect accuracy. The database reconstruction still got the great majority--nearly 80%--of these cases wrong. This may be partly because Summary File 1 P012A-I only gives five-year groups and the database reconstruction had to guess exact age, or it may reflect the efficacy of traditional disclosure control for these very small blocks. In any case, the error rate on tiny blocks is too high to make the data very useful for reidentification. Nevertheless, the Census Bureau probably ought to get rid of the tiny blocks by merging them with larger blocks. Especially since the Census Bureau has gotten rid of swapping, tiny blocks may pose a disclosure risk.
Hullman notes that "on Twitter, Ruggles states that the Census Bureau 'has reluctantly acknowledged' that 'an outside intruder would have no means of determining if any particular inference was true' ... Ruggles' assertion about the Census admitting there's no way doesn't seem quite right."
As we indicated in the research brief, this statement is based on a blog post from Acting Director Ron Jarmin, who wrote: "The accuracy of the data our researchers obtained from this study is limited, and confirmation of re-identified responses requires access to confidential internal Census Bureau information ... more than half of these matches are incorrect, and an external attacker has no means of confirming them."
As Hullman notes, John Abowd disputes his boss, suggesting that an outside attacker could do field work to assess the accuracy of the purported reidentification. That is theoretically possible, but as soon as the private investigator realized that most of the reconstructed data are incorrect, they would give up the pointless exercise.
Hullman writes that she does not understand our analogy to a clinical trial. She comments "It's as though Ruggles and Van Riper want to be comparing results of a reconstruction attack made on differentially private versions of the same 2010 Census tables to non-differentially private versions, and finding that there isn't a big difference." I have no idea where this is coming from. We do not evaluate differential privacy or the TopDown algorithm and make no reference to it except to cite the work of others who have analyzed the impact of these approaches on census accuracy.
Our paper is about database reconstruction and reidentification, not differential privacy. We find that there is little difference between the reconstructed data and a null model of random assignment of age and sex together with a simple assignment rule for race and ethnicity. This means that even if the reconstructed data sometimes matches the characteristics of an actual person, it does so by chance, not because of the magic of 6.2 billion statistics, simultaneous equations, and Gurobi. We can't evaluate the efficacy of database reconstruction just by counting the number of matches with real census data; to assess the threat, it needs to be compared against a null model of random guessing.
When it comes to reidentification, we point out that just because 38% of individuals in a commercial database match someone enumerated by the census, that is meaningless without a null model. To evaluate the risk of reidentification we need to know how much better the "reconstructed" individuals match than do individuals randomly selected from the commercial database. Unless the Census Bureau can show that the database reconstruction increases the likelihood of finding the person, the exercise is pointless.
Hullman concludes "Bottom line is that showing that a more random database reconstruction technique matches fairly well in aggregate does not invalidate the fact that the Census reconstructed 17% of the population's records." Basically, she is conceding that the much-vaunted database reconstruction and reidentification experiment does not perform much better than a roll of the dice. It sounds like randomly guessing someone's characteristics and sometimes guessing correctly is considered by Hullman to somehow be a disclosure risk. I guess we had better think about banning random number generators.
Finally, Hullman titles her blog post "Shots taken, shots returned regarding the Census' motivation for using differential privacy (and btw, it's not an algorithm)" and she closes with a disparaging comment suggesting that my testimony in the Alabama case described differential privacy as an algorithm. In fact, that is incorrect.
The Census Bureau often does treat DP as if it is an algorithm. For example, Acting Director Ron Jarmin wrote "We are deploying differential privacy, the gold standard for privacy protection in computer science and cryptography, to preserve confidentiality in the 2020 Census and beyond." I avoided that language, writing "Implementation of differential privacy generally involves calculating cross-tabulations from "true" data and injecting noise drawn from a statistical distribution into the cells of the crosstabulation." My only reference to the term "algorithm" was to the post-processing algorithm. Before posting, maybe Hullman should have checked the testimony instead of just quoting Abowd's unfounded attack on my qualifications.